
AI in Vivo
AI in Vivo is a nonprofit foundation built to combine machine learning with scientific research.
Elements
Researchers in various fields have separately discovered the elements needed to enable the integration of AI with scientific discovery, simulation, and technology development. AI in Vivo is working to combine these elements into a software engine at the foundational level.
The information entropy of a system can be used to measure its fitness. This is important for ecosystem health.
02. Multiscale Modeling
Different physics, variables, and processes are relevant at different lengthscales and timescales.
03. Structural Learning Methods
Statistical methods, such as Bayesian belief networks and hidden Markov models, in addition to machine learning methods, such as MIT's AI Physicist, can be used to infer the hierarchical composition of systems and unknown variables.
04. Design of Experiments
This allows for reductions in model uncertainty, improvements in engineering design, and the diagnosis of problems based on symptoms.
05. Modularity
The modular design of simulation objects allows for computational efficiency and dynamic course-graining and fine-graining.
06. Continuous Improvement
Methods to improve model accuracy and simulation speed include the optimization of information optimization, AI theorycrafting, and unification of models in different regimes.
07. Generative Capability
In addition to the deduction of models for existing systems, identical processes can be used to design new systems with desired properties.


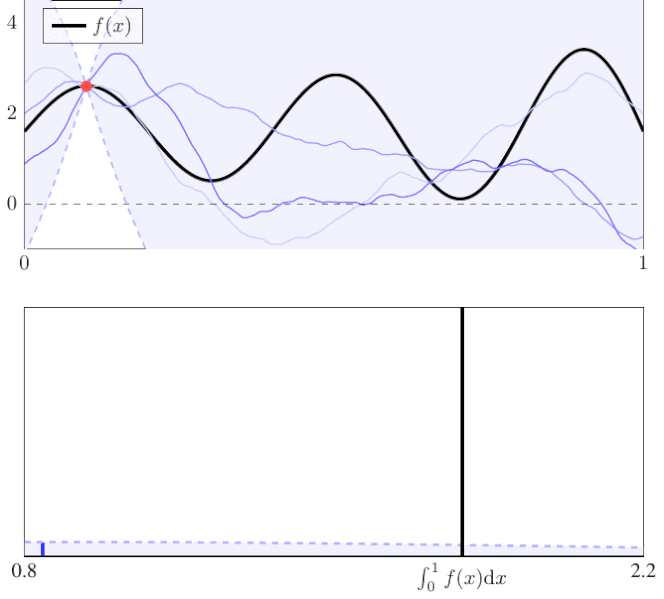
Universal Simulation and Modeling Engine (USME)
-
This is an object-oriented programming engine that's also dynamic, meaning the objects are able to modify themselves dynamically in order to optimize their entropy vs. accuracy ratio.
-
Items 1-7 in Elements are various features of this technology.
-
The concept of "object" is considered to be foundational, similar to the concept of "set" in number theory. This makes the engine domain-agnostic, which means it could be used for climatology, chemistry, medicine, or other scientific fields.
Design of synthetic cartilage tissue using reinforcement learning
-
There are approximately 400,000 total hip replacement surgeries and 700,000 knee replacement surgeries performed in the US each year.
-
The goal is to develop a synthetic cartilage replacement tissue using Reinforcement Learning in combination with molecular simulation.
-
A synthetic cartilage tissue must have high tensile and compressive strength, high durability, high lubricity, anti-fouling properties to prevent to the immune system Foreign Body Response (FBR), resistance to biodegradation caused by free radicals and proteolytic enzymes, and the ability to integrate with the adjacent bone surface.
Study of community and climate health
-
The interactions among social, industrial, and environmental processes are complex. It's insightful to measure these connections accurately through the lens of state entropy.
-
Since money is the social reward metric, if the value of currency is modified to include these interactions, what effect does it have on individual well-being, economic activity, and ecosystem health?
-
Many climate models are unable to interact with each other. A multi-model unification framework can make these simulations more robust. Optimization through course-graining and fine-graining can increase computational efficiency.

Projects

Very Long-term Possibilities
-
In the long-term, the possibility exists to destroy individual cancer cells, rebuild cells in the nervous system, and make other highly specific and individualized cellular interventions. The development of treatments for medical conditions is difficult and time-consuming due to complex interactions present in the body and the obstacles associated with identifying cellular mechanisms of action, which are often unknown to medical researchers.
-
There are potential applications in the entertainment industry, particularly video games. In one example, the game company Ubisoft has pioneered several fast-physics-learning techniques to improve player experience of in-game physics. It’s also possible that object transformations can be experienced subjectively as narratives, which brings the historically difficult problem of automated narrative generation into the realm of possibility.
-
Potentially groundbreaking applications exist in the fields of engineering design. If reinforcement learning is combined with complex system analysis, it produces the ability to design systems across multiple length- and timescales. This creates the potential to generate complex engineering systems with an effectiveness beyond the capability of human experts.
-
Other possible applications exist in chemistry and materials science. The ability to correlate molecular structure to macroscale chemical and material properties, and to design chemicals and materials with desired properties, is a major unsolved problem..
About
Story
AI in Vivo was founded by Stephen Casey, an engineer at the NASA Langley Research Center.
Technology
There's a need in the research community for a Universal Simulation and Modeling Engine (USME) that observes information entropy and is highly object-oriented, dynamic, and expressive.
Vision
Proposed projects using this technology include the design of synthetic cartilage tissue using reinforcement learning, and the study of economic and climate system health.

Contact
Potential collaborators and supporters, send a message below.